Actuator noise is a significant challenge in robotics, where mechanical actuators—the components responsible for generating movement—often produce unwanted noise or disturbances. This noise can affect the performance and accuracy of robotic systems, leading to degraded control, reduced precision, and overall inefficiency. In environments where precision is paramount, such as medical robotics, manufacturing automation, and autonomous vehicles, minimizing actuator noise is crucial to ensure that the robot’s actions are as intended.
MHTECHIN, an advanced AI-driven platform that excels in real-time decision-making, adaptive learning, and system optimization, offers an innovative solution for actuator noise compensation. By integrating machine learning algorithms with sophisticated sensor feedback and control systems, MHTECHIN enables robots to detect, predict, and mitigate actuator noise in real-time, thereby improving performance and precision.
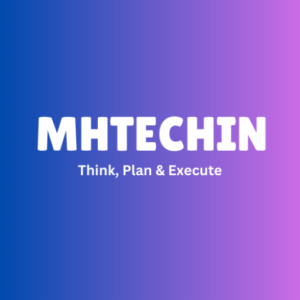
In this article, we will explore how AI for actuator noise compensation can be implemented with MHTECHIN, and how it transforms robotic systems by ensuring greater accuracy, reliability, and operational efficiency.
1. Understanding Actuator Noise in Robotics
An actuator in a robot is responsible for converting electrical energy into mechanical movement, whether it’s a DC motor, hydraulic piston, servo, or pneumatic system. However, actuators are prone to generating noise or disturbances that can significantly impact the robot’s behavior. This noise is caused by factors such as:
- Mechanical imperfections: Friction, backlash, and wear-and-tear in the actuator components.
- Control system errors: Uncertainties or errors in the feedback loop during the operation of the actuator.
- Environmental disturbances: External factors such as temperature variations or external forces that affect actuator performance.
- Electromagnetic interference: Noise generated by electrical components or signals in proximity to the actuator.
Actuator noise can manifest in various ways, such as jerky movements, delayed responses, positioning errors, or vibration. In precision tasks like robotic surgery, industrial assembly, or mobile navigation, even small deviations caused by actuator noise can be critical.
Types of Actuator Noise:
- High-Frequency Noise: Often due to motor vibrations or switching disturbances, affecting the smoothness of the actuator’s movements.
- Low-Frequency Noise: Caused by mechanical misalignments or gradual wear, which can cause drift or delayed response.
- Quantization Noise: Introduced by digital control systems, where the signal is discretized, leading to rounding errors.
2. How MHTECHIN Can Address Actuator Noise
MHTECHIN enhances actuator noise compensation by using advanced AI algorithms, particularly machine learning and adaptive control techniques, to model and predict noise patterns and adjust the robot’s actions accordingly.
a. Real-Time Noise Detection and Characterization
To effectively compensate for actuator noise, it is essential to first detect and characterize the noise. MHTECHIN‘s real-time data processing capabilities enable robots to monitor and analyze actuator signals continuously. By analyzing the feedback from force sensors, accelerometers, and encoders, MHTECHIN can identify anomalies or patterns indicative of noise.
- Example: In a robotic arm performing precise assembly tasks, MHTECHIN can monitor the forces and positions reported by the arm’s sensors and detect fluctuations or irregularities in actuator behavior. If noise is detected, MHTECHIN can then adjust the robot’s control system to correct for these deviations.
b. Predictive Noise Compensation
Once actuator noise is detected, MHTECHIN uses its predictive models to anticipate future noise patterns based on historical data. Using machine learning techniques like regression analysis, time-series forecasting, and deep learning, MHTECHIN can build an accurate model of the actuator’s behavior under different noise conditions.
- Example: In medical robotics, where precision is critical, MHTECHIN could model the noise produced by a robotic surgical arm’s actuators. Using this model, it can predict and compensate for future noise disturbances in real-time, ensuring that the arm follows the desired path with high precision.
c. Adaptive Control for Noise Mitigation
One of MHTECHIN’s core strengths is its adaptive control system, which continuously adjusts control parameters to compensate for varying levels of actuator noise. This allows the robot to modify its motion commands in response to changing noise levels, optimizing performance without needing explicit reprogramming.
- Example: In autonomous vehicles, actuators responsible for steering, braking, or suspension systems can experience noise due to terrain irregularities. MHTECHIN can dynamically adjust the control inputs to the vehicle’s actuators to counteract the impact of the noise, ensuring that the vehicle follows the desired path with minimal deviations.
d. Sensor Fusion for Enhanced Noise Compensation
MHTECHIN can leverage sensor fusion to combine data from multiple sources (e.g., accelerometers, gyroscopes, encoders, and force sensors) to improve the accuracy of actuator noise compensation. By integrating this data, MHTECHIN can create a more robust model of the system’s dynamics, better distinguishing between actual motion and noise disturbances.
- Example: For a robotic manipulator working in a factory setting, sensor fusion can combine encoder data with force feedback from the tool being used. This allows the system to identify when noise is present in the actuator and adjust the force applied to objects, ensuring precise operations even in noisy conditions.
3. Applications of AI for Actuator Noise Compensation with MHTECHIN
The integration of AI-driven noise compensation with MHTECHIN has transformative potential in many robotics applications. Below are a few key areas where this technology can be applied:
a. Robotic Surgery and Precision Medicine
In robotic surgery, the accuracy and reliability of actuators are crucial to ensure the safety of patients. Even minute deviations caused by actuator noise can lead to errors in incision, positioning, or tool manipulation.
- Example: A robotic surgical system used for minimally invasive surgery can rely on MHTECHIN to continuously compensate for any actuator noise, allowing the robot to make precise, millimeter-scale adjustments during surgery, even in the presence of environmental or mechanical noise.
b. Industrial Robotic Arms and Automation
In industrial automation, robotic arms are often used for precision tasks such as welding, assembly, or painting. Actuator noise can reduce the precision of these tasks, leading to defects or inefficiencies.
- Example: An industrial robotic arm performing spot welding can use MHTECHIN to minimize noise in the actuator responsible for controlling the welding torch’s movement, ensuring that the weld is placed accurately and without defects despite potential mechanical noise or vibrations.
c. Autonomous Vehicles and Drones
For autonomous vehicles and drones, actuator noise can affect the robot’s ability to control speed, direction, or orientation, leading to erratic behavior or errors in navigation.
- Example: An autonomous drone navigating in a windy environment can use MHTECHIN to compensate for actuator noise in its motors and propellers. By predicting and adjusting for noise disturbances, the drone can maintain stable flight and accurate navigation despite external environmental factors.
d. Assistive Robotics and Prosthetics
In assistive robotics, such as robotic prosthetics or exoskeletons, actuator noise can interfere with the smooth and precise movement of the device. For users with disabilities or mobility impairments, even slight errors can have a significant impact on functionality and comfort.
- Example: A robotic prosthetic arm can leverage MHTECHIN to minimize noise in its actuators, ensuring smooth and controlled movement, particularly when adjusting for fine motor skills or when interacting with delicate objects.
e. Agricultural Robotics
In agricultural robotics, precision is critical for tasks such as harvesting, planting, or soil monitoring. Actuator noise can impact the accuracy of these tasks, especially in dynamic or unpredictable environments like fields or greenhouses.
- Example: A robotic harvester in a crop field could use MHTECHIN to compensate for actuator noise from the system’s robotic arms. By predicting noise caused by soil interaction or crop resistance, the system can adjust its movement and force application to ensure optimal harvesting without damaging the crops.
4. The Future of Actuator Noise Compensation with MHTECHIN
The future of actuator noise compensation with MHTECHIN is highly promising. As robotic systems continue to evolve and become more complex, the need for real-time, adaptive compensation techniques will grow. With the capabilities of AI-driven platforms like MHTECHIN, robots will be able to operate with unprecedented precision and reliability, even in environments with significant noise disturbances.
Future Directions:
- Advanced noise prediction models based on deep learning, enabling even more accurate anticipation and compensation for actuator noise.
- Cross-robot noise compensation, where robots can share noise patterns across a fleet of machines, learning from each other to improve performance globally.
- Integration with other AI systems such as
vision systems and predictive maintenance, leading to a fully integrated robot with zero-impact actuator noise.
In conclusion, AI for actuator noise compensation with MHTECHIN is a game-changing approach that improves the accuracy and reliability of robotic systems across industries. Whether in surgery, industrial automation, or autonomous navigation, the ability to compensate for actuator noise ensures that robots can perform tasks with the precision required for high-stakes applications. The combination of real-time feedback, predictive learning, and adaptive control paves the way for more efficient, robust, and reliable robotic systems in the future.
Leave a Reply