Neural plasticity is a fundamental property of the brain that enables it to adapt to new information, recover from injury, and optimize behavior based on experience. This concept, often referred to as neuroplasticity, is central to learning, memory, and cognitive functions. In the context of artificial intelligence (AI) and machine learning (ML), neural plasticity can be emulated in neural networks to enhance their adaptability, robustness, and learning efficiency.
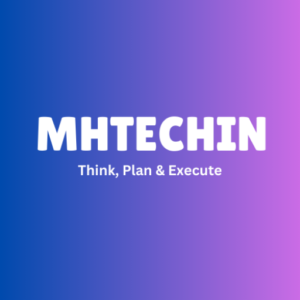
MHTECHIN, an advanced AI platform known for its adaptive learning, real-time feedback integration, and multimodal capabilities, provides an ideal environment to integrate the concept of plasticity into AI systems. By mimicking the adaptability and learning processes seen in biological systems, MHTECHIN facilitates the creation of highly flexible, self-improving neural models that can respond to changes in data, environments, or tasks with minimal retraining.
This article will explore the concept of plasticity in neural models, how MHTECHIN incorporates this principle, and the transformative impact this can have on the development of adaptive AI systems in various industries.
1. What is Neural Plasticity?
Neural plasticity refers to the ability of neural networks—whether biological or artificial—to reorganize themselves in response to changes in input, experience, or environmental conditions. This plasticity enables the brain to continuously adapt by rewiring synaptic connections, strengthening or weakening certain pathways, and learning new patterns of behavior or information.
Types of Plasticity:
- Hebbian Plasticity: Often summarized as “cells that fire together, wire together.” This form of plasticity strengthens the connections between neurons that are activated together, leading to improved learning and memory.
- Homeostatic Plasticity: Helps maintain overall balance within neural networks by adjusting the strength of connections to avoid over-excitation or under-excitation of neurons.
- Structural Plasticity: Involves the physical reorganization of neural circuits by adding or removing synapses or dendrites in response to learning or environmental changes.
- Experience-Dependent Plasticity: Refers to the changes that occur in neural networks as a result of environmental experiences, sensory input, or novel tasks.
In artificial neural networks, plasticity is similarly crucial for enabling networks to adjust to new data, recover from errors, and fine-tune their weights and connections to improve overall performance.
2. Plasticity in Neural Models and Its Importance
In artificial neural networks (ANNs), plasticity manifests as the ability of the network to update its weights and activation functions in response to new data or changing environments. Incorporating plasticity principles into neural models helps address challenges like overfitting, underfitting, and catastrophic forgetting (where networks forget previously learned information when exposed to new data).
The importance of plasticity in neural models lies in:
- Adaptability: Neural models with plasticity can adapt to new, unseen data without the need for full retraining. This is particularly important in dynamic environments, where data and conditions constantly change.
- Lifelong Learning: Plasticity allows neural networks to learn continuously over time without forgetting old knowledge, enabling systems to acquire knowledge from diverse experiences.
- Robustness: By adjusting to new stimuli or disruptions, networks with plasticity can recover more effectively from unexpected changes or disturbances in the environment.
- Energy Efficiency: Plastic networks can optimize resource allocation by dynamically adjusting the intensity of connections between neurons, reducing computational overhead.
3. How MHTECHIN Enhances Neural Plasticity
MHTECHIN enhances the capabilities of neural models by embedding plasticity principles into its architecture. The platform incorporates several advanced features that enable AI systems to exhibit adaptive learning, including real-time feedback, dynamic weight updates, and self-organizing structures.
a. Real-Time Learning and Feedback Integration
One of the core strengths of MHTECHIN is its ability to integrate real-time feedback. This means that neural models on MHTECHIN are constantly updating and adjusting based on live data, allowing them to modify their weights and structures on-the-fly. This mirrors the brain’s ability to adapt to sensory input and make adjustments to its behavior in real time.
- Example: In autonomous driving, MHTECHIN-powered systems can adjust the decision-making process based on real-time sensor input. If an unexpected obstacle is detected, the AI can modify its trajectory or control signals immediately, ensuring smooth operation.
b. Continuous Learning (Lifelong Learning)
Unlike traditional machine learning systems that require retraining from scratch as new data becomes available, MHTECHIN allows neural models to engage in lifelong learning. By using plasticity-inspired algorithms, the system can continuously integrate new knowledge without forgetting previously learned patterns. This ensures that AI models stay up to date with evolving environments and data.
- Example: In a healthcare diagnostic system, MHTECHIN can continuously learn from new patient data, adjusting diagnostic models based on evolving medical trends or emerging diseases, without erasing knowledge from past cases.
c. Dynamic Weight Adjustment and Self-Organization
At the heart of plasticity in neural models is the ability to adjust the weights between neurons based on experience. MHTECHIN utilizes advanced weight modulation techniques, including Hebbian learning and homeostatic plasticity, to enable the neural network to adjust its internal representations dynamically.
- Example: In robotics, a robotic arm powered by MHTECHIN can fine-tune its movement strategies based on sensory feedback. If an obstacle is encountered, the robot can adjust its motor commands to find the most efficient path without human intervention.
d. Error Correction and Self-Healing
Plasticity allows neural models to learn from their mistakes and correct errors over time. MHTECHIN integrates error correction algorithms inspired by biological neural plasticity, enabling AI systems to recover from mistakes and improve their performance based on feedback. This is particularly valuable in systems that need to operate autonomously or in complex environments.
- Example: In predictive maintenance systems, if a machine’s failure is misdiagnosed or the system predicts a failure too late, MHTECHIN can adjust the model’s parameters to improve its predictions for future failures, based on the error.
4. Applications of Plasticity in Neural Models with MHTECHIN
The integration of plasticity into neural models through MHTECHIN has broad applications in various fields, where adaptability, learning, and robustness are critical.
a. Autonomous Systems and Robotics
Plasticity in robotic systems allows robots to continuously improve their performance based on interactions with the environment. MHTECHIN enables robots to adjust their movements, strategies, and behaviors in real-time, allowing them to perform complex tasks more efficiently.
- Example: In warehouse robotics, MHTECHIN-powered robots can adapt to changes in inventory layout and dynamically adjust their navigation strategies without requiring constant human intervention.
b. Healthcare and Medical Diagnostics
In medical diagnostics, plasticity allows AI systems to continuously learn from new data, medical research, and evolving healthcare conditions. MHTECHIN can help develop systems that adapt to new diseases, treatment protocols, and patient-specific data, improving diagnostic accuracy over time.
- Example: AI-powered health monitoring systems can dynamically adapt to a patient’s condition, adjusting recommendations and treatment plans as new data is collected.
c. Natural Language Processing (NLP)
Plasticity in NLP models helps them adjust to new language patterns, slang, or cultural nuances over time. MHTECHIN enables NLP systems to continually improve their language models through ongoing training with new datasets, including evolving trends in language usage.
- Example: A virtual assistant powered by MHTECHIN could continuously adapt to user interactions and preferences, improving its ability to understand and respond accurately to different language forms and contextual cues.
d. Financial Systems and Risk Management
In financial systems, plasticity can enhance predictive models used in fraud detection, stock market analysis, and risk management by allowing them to adjust to new patterns of behavior or financial crises.
- Example: MHTECHIN can power predictive models that learn from market fluctuations, detecting emerging financial trends and adjusting investment strategies accordingly.
e. Personalized Education Systems
Adaptive learning systems that use plasticity can tailor educational experiences to the individual needs of students. MHTECHIN can help create intelligent tutoring systems that continuously adjust the learning path based on real-time student performance, improving learning outcomes.
- Example: An AI tutor powered by MHTECHIN could continuously adapt its teaching methods based on the student’s progress, learning style, and understanding, ensuring personalized and efficient learning experiences.
5. The Future of Plasticity in AI with MHTECHIN
As AI systems become more complex and integrated into real-world applications, the need for adaptive, lifelong learning becomes increasingly important. MHTECHIN’s incorporation of neural plasticity principles paves the way for more intelligent, robust, and efficient systems that can learn continuously and adapt to new challenges without needing extensive retraining.
The future of plasticity in neural models with MHTECHIN holds exciting possibilities:
- Enhanced Human-Machine Collaboration: Machines that can adapt based on human feedback in
real-time, making collaborative work more efficient and intuitive.
- Self-Improving AI: Neural models that continuously evolve and improve based on their experiences, leading to AI systems that are always learning and adapting.
- More Resilient Systems: AI systems that can dynamically adjust to disruptions, errors, or changing conditions, ensuring they remain effective in unpredictable environments.
In conclusion, plasticity in neural models powered by MHTECHIN represents a significant advancement in the field of AI, enabling systems to adapt, learn continuously, and recover from errors in real time. By combining biological-inspired learning with cutting-edge technology, MHTECHIN fosters the creation of AI systems that are not only more intelligent but also more adaptable, efficient, and capable of operating in complex, dynamic environments.
Leave a Reply